AI systems are regarded as intelligent if they can perform tasks that would usually require human intelligence. These include general capabilities such as solving problems, recognizing patterns, understanding language, and even making decisions. Depending on complexity, AI may have primitive systems that execute pre-defined steps or advanced systems that involve reasoning, perception and learning.
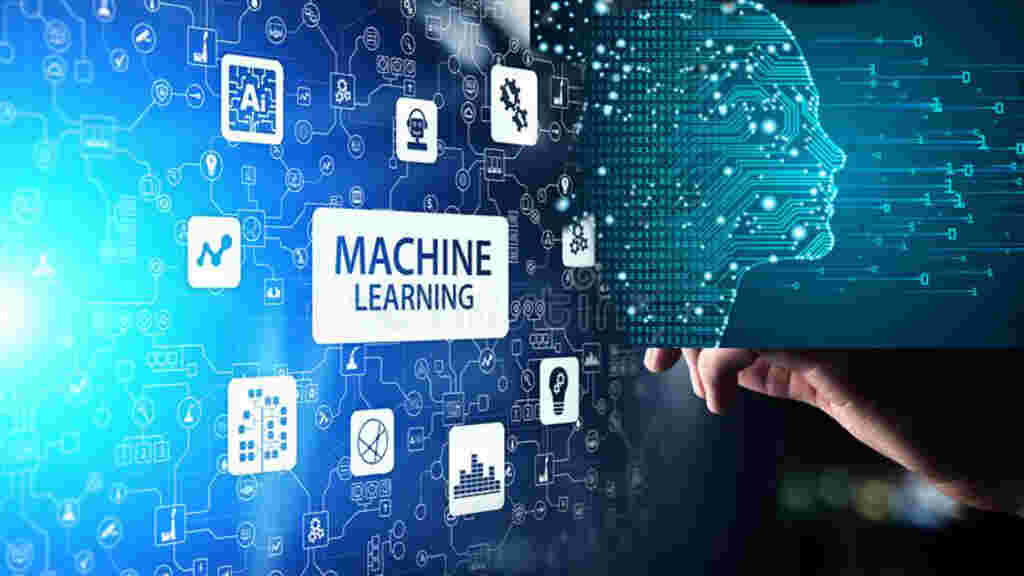
Table of Contents
AI Overview
Development of AI
The journeys of Artificial Intelligence or AI began with scientists thinking of machines as “thinking” machines in the mid-20th century era. The birth of artificial intelligence is credited to 1956, during the Dartmouth conference where researchers first coined the term “Artificial intelligence”. The first works on AI were limited to symbolic logic and rule learning, and because of the computer power available then, development was very slow.
The 1980s saw a resurgence of interest, facilitated by the emergence of development of machine learning science and artificial neural networks. However, it was not until the beginning of the twenty-first century, with the development of technologies for the collection and processing of big data that AI started developing quickly. AI is now an active area of research that interfaces mathematics with computer science, cognitive psychology, neuroscience as well as linguistics.
Core Concepts and Components of AI
AI has a number of sub-fields, each of which has its own set of practices and application:
Machine Learning
Machine learning (ML) is a subfield of AI which focuses on algorithms which enable a computer to evolve through “learning” from a particular set of inputs over time without necessarily being hard-coded. Image recognition, recommendation systems or even predictive analytics fall under ML applications.
Deep Learning
Deep learning, a subset of DL, applies neural networks with multiple layers to detect hierarchical patterns within large data sets. Deep learning, as the name suggests, is based on how deep learning about various tasks such as voice, language, and images can be done by a human brain structure, relieving a number of tasks.
Natural Language Processing (NLP)
NLP provides capabilities to computers regarding interaction and understanding human languages, interpretation or generation. Applications of such technologies range from chatbots or virtual assistants to machine translation or mood detection by inference.
Computer Vision
Computer vision is an application of AI enabling the computer to understand picture and video data received from the outside world. It is used in facial recognition, self-driving cars, and analyzing medical images.
Robotics
Robotics is the science and technology which possesses both Artificial Intelligence and engineering in order to develop machines which can undertake varying tasks on their own or with some assistance or user direction. Areas of ai that hold relations with robotics are; self-driving cars, drones and surgical robots.
Types of AI
An AI can be differentiated from its functions and possible uses:
Narrow AI
Narrow AI, or weak AI refers to systems that are designed and trained for a specific task like voice recognition systems, recommendation systems, etc. At present, the majority of AI applications fall under the domain of narrow AI and are able to perform very well for specialized tasks, but do not have general intelligence.
General AI
General AI, also referred to as strong AI, is a set of concepts around machines\’ abilities that are close to, or maybe even superior to, humans in solving a wide range of tasks and adapting to a variety of situations. There are no such machines at present and General AI as such still remains theoretical and has no examples which are known to exist at the current time.
Superintelligent AI
Such an AI system would know far more than a human being and will have far superior problem solving and creative abilities. Superintelligent AI is, however, hypothetical, but it generates a great deal of concern regarding ethics and even the very existence of humanity.
Key Techniques in AI
As the goal that the specific AI application is designed for differs, so do the methods that it employs:
Supervised Learning
In supervised learning, models are taught to focus on labeled data with an input-output scenario. That process has also a wide scope of application, especially in spam detection or image classification.
Unsupervised Learning
There is no label attached to the data in an unsupervised learning technique hence the focus is on finding previously unknown patterns without any direction. Anomaly detection and customer segmentation make use of clustering and association techniques.
Reinforcement Learning
Reinforcement learning refers to progress that occurs via agents, interacting with the environment around them and rewarded/punished for their actions. It works particularly well in any sequential decision making problems as found in robotics and the artificial intelligence of games.
Neural Networks and Deep Learning Architectures
A neural network is comprised of numerous nodes, or “neurons” which share the same structure composition as the human brain. Deep neural networks employ a stacked architectural configuration, such as convolutional or recurrent neural networks, which are important to AI advancements, to extract information from more complicated structures.
Transfer Learning
Unlike building a model from scratch, transfer learning enables AI models to use knowledge obtained from one area into another area avoiding the need for large volumes of data. It is especially helpful for scenarios with small volume of labeled data.
AI in the Real World
There are numerous industries whereby AI has and will make a huge impact:
Healthcare
Healthcare is another field that is having enormous positive effects due to AI, with medical assistance in diagnostics, drug developments, and creating personalized treatment approaches. For example, deep getting- images algorithms can be as accurate as a medical doctor. Predictive models can identify patients who are prone to certain diseases.
Finance
AI is advancing fraud prevention, trade algorithms and virtual assistant customer service in finance. AI technologies are being utilized to provide investment managers and banks information on trends as well as assisting in analyzing risks and making objective decisions.
Retail
Retailers are embedding AI to create targets for marketing campaigns, manage stock levels and set prices by the hour. AI-powered marketing analytics enhance customer experience helping boost sales and customer satisfaction.
Transportation
AI in transportation is responsible for the development of driverless cars, traffic forecasting, and fleet management systems. Companies such as Tesla or Waymo are the developers of the self-driving technologies that are expected to enhance safety and efficiency of the roads.
Ethics and Challenges in AI
The development of AI raises big ethical and social issues that cannot be ignored.
Bias and fairness
AI systems inherit problems of biases originating from the training data, therefore producing unfair and often discriminatory results. Bias in AI has been noted in several areas such as wedding, law enforcement agencies and even health care systems, thus it is important to have a comprehensive system of equitable and ethical AI implementation.
How to resolve failed to pull Helm chart
Privacy concerns
With Artificial Intelligence being a data-driven technology, it causes issues of privacy and surveillance especially from the AI-enabled technologies that are rapidly transforming or redefining today’s society. Technologies like facial recognition or data tracking go beyond the boundaries of personal freedoms and must be regulated in order to protect the rights of society.
Economic Displacement Social AI is predicted to make automation with a negative impact on job opportunities. Manufacturing industries and customer support centers will be the industries most likely to experience job loss. AI itself creates new job roles however there is an immediate urgency for reskilling and economic restructuring as well.
Security Risks Threats to security are also introduced by AI such as an abuse of AI(e.g deep fakes, free-roaming weapons systems) and an abuse of AI systems with extant vulnerabilities. The need of the hour is to guard the already built AI systems by making them machine and human proof.
Future of AI The future does bring a lot of promise of AI but also calls for prudent stewardship. Adjacent areas such as quantum computing and explainable AI (XAI) is not only expanding the frontier of AI but also trying to fill the gaps in the current paradigm.
Quantum AI: Optimization problems are mainly the hardest hit areas by quantum computing’s goal of accelerating current reaching AI processing
Explainable AI(XAI): AI systems will only grow more complex in the future so it is crucial AI systems have an interpretable facet. In order to build trust in AI power decisions, XAI focuses on convincing users AI models are not what they seem.
Human-AI Collaboration: The focus should be on systems collaborating with AI where humans direct the process and ideas, while AI performs computation and other minor tasks.
Policy and Regulation: Countries and institutions across the globe are struggling with the question of how to regulate AI so as to promote creativity while minimizing the risks it poses to humanity.
Conclusion
AI is a diverse area with a great future ahead. It has been said that AI has a plethora of practical uses, but it also comes with some ethical and security risks. Having knowledge of AI’s key concepts, its various kinds, different methods and problems can help an individual understand the scope of AI’s application in the society. Great caution should be exercised in future as AI continues to grow- adopting the good whilst at the same time shielding against the evil.