AI environments are parenting AI agents to photograph their environment and respond and work with it. Data may be in intercepts by penses like cameras or microphones.
After the decisions have been reached, the inner mind mіkі, logic, and analyses and their inner picture of that time and place and decision are executed
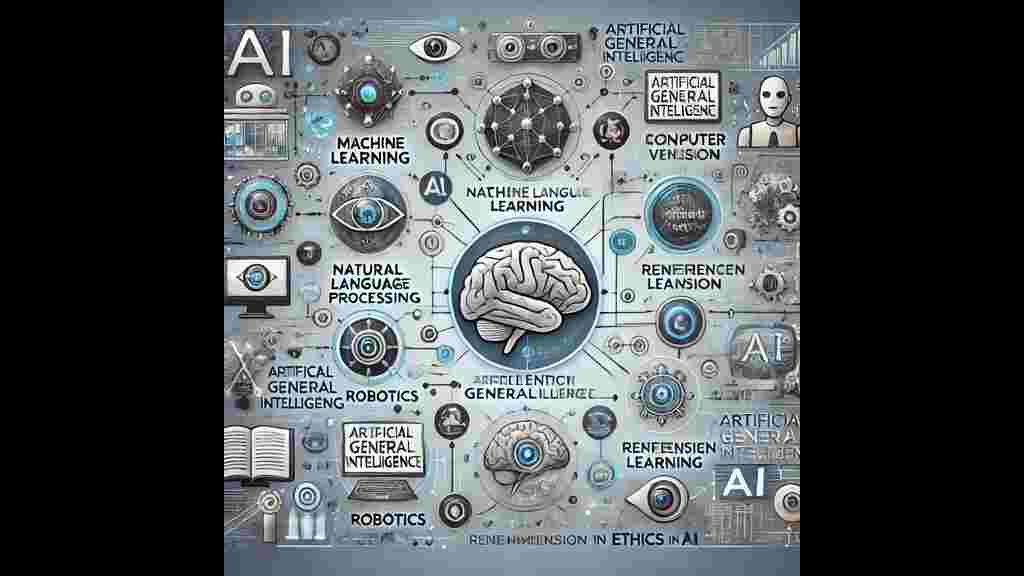
Table of Contents
AI Agents and Enviroments
Human-Agent Interactions
Because AI is becoming a bigger part of people’s lives and industries, the design and analysis of the interactions between human and machine agents have become subjects of primary attention. To further this endeavor, a number of parameters such as reactivity, proactivity, social, and learning which AI agents o best designed them should be integrated into the interactions. They provide reactivity and adaptiveness that allows them to perform monotonous functions, improve service delivery, offer mental health services, or conduct interviews, among other things.
An agent is a soft ware that has sensors to observe the environment and actuators to perform action on the environment. In AI, they are agents that serve a task as intelligent helpers and built from Natural Language Processing and Machine Learning enabling them to answer user requests, retrieve information and make queries to other systems, or teach themselves based on what they did before or what feedback they received.
Learning Layer Models (LLMs) allow the agents to understand what the user wants and to act accordingly. That works best for chatbots which are based on generative and continuously learn to address the needs of the specific user.
AI agents are represented by e-commerce engine recommendations, navigational systems and fraud detection systems, among others. For instance, even a simple LLM could be trained to locate the quickest route that would make optimal use of fuel while avoiding lengthy waits in traffic and paying tolls.
AI agents are a part of automation and robotics – “soft” robots simulating living forms or “swarm intelligence” imitating the behavior of insects are already commonplace in surgery, transport, or space missions. This area, however, remains very dynamic, incorporating VR or AR technologies, which have already become the basic standards in everyday life.
There are a lot of ways AI agents can be game changing. They can transform business processes as well as the way that customers interact with companies. But it is worth pointing out that there is no uniform code of ethics in ai agents; thus in order to perform impactful actions, there should be a human approval in advance which guarantees the ethical, legal, and societal responsibilities while preventing any possible negative effects.
Robotic Agents‘ The last chapter on “Agents”, covers the concept of AI virtual and physical agents. With machines, AI can exist physically, like factory robots and operationally through chatbots. It’s worth pointing out that AI and algorithmic agents learn and build a capability with time to continually enhance performance Several urelements encompassing AI. AI has sensors and actuators, with the addition of having brains or decision-making. Sensing is the work of sensors, all actions are taken by actuators. In terms of decision-making tools used in machine learning, automation which sometimes incorporates deliberative models, reactive models or both. It deals with how AI agents deal with problems.
There are AI and robotic agents that are useful in the aviation industry in controlled environments and help automate complicated processes that human beings could not perform alone. AI tasks of robots include dealing with difficult materials that AI is able to easily manage in a manufacturing environment that employs algorithms and computer vision to perform tasks such as welding and painting more efficiently and accurately than humans. AI and robot systems will also enable individuals with physical integration systems to perform autonomously in an average home by assisting them with self-maintaining tasks.
Adding a learning module to the architecture of AI agents is one more solution that can allow them to improve their efficiency over time, as this would be augmenting the AI agent’s knowledge of its surrounds with time. Such feedback might either emanate from its critics who can be human operators or other AI agents assessing performance, or even its environment. One claim the authors are able to justify is that an agent critic could help in focusing (engineers) on evaluation and providing explanations whereas the environment could receive information on what the agent has done.
There are also various systems found in other industries and applications. From ensuring that transport systems networks are optimized, in reducing energy consumption in smart buildings or houses, providing security in financial services industry, in monitoring patients while they undergo personalized health care delivery systems, increasing efficiency in supply chains and logistics, or in the provision of intelligent adversaries in computer games, enhancing the operation of various user interfaces – there are uses for intelligent systems on-premise, in the cloud as well as mobile platforms.
Model-Based Reflex Agents
As a consequence, model-based agents are distinct from simple reflex agents, as within any of their reactive actions, they generate a mental representation/model of their current environment and utilize it to anticipate successive states of that environment based on their current perception or any subsequent relevant information that may be available thereafter.
When you are a model-based reflex agent, you follow a model and decide which action takes priority. The model that they make use of can, for instance, help them detect dirt which leads them to clean dirt in a room, where their percepts correspond with parameters predetermined rules as to what actions can be undertaken (like an “IF…THEN…” list) that displays the action possibilities. Naturally, such situations exist when the agents don’t have complete information and, while such a model may work efficiently, there will be some drawbacks going with this model.
An example of a model-based reflex agent is an automatic air conditioning system that compares the actual and desired temperature settings in a room before switching on air conditioning. Such systems can save energy which in turn saves the cost of energy bills.
In feature, Model based agents are Self driving cars that build an internal understanding of the traffic and road conditions, which gets updated through external stimuli -like rain which can fall on the model (the sensor on the car) itself. Model-based reflex agents are important for managing intelligent transport systems, and for Instrumental Automation Systems to help predict expected machine or material performance & availability and operate accordingly.
There seems to be a growing reliance by businesses on artificial intelligence as technology that would be able to perform various tasks and deliver healthcare and many other services effectively. It is clear that these systems can assist us with accomplishing all sorts of tasks ranging from answering phone calls to writing texts, uploading data files, creating visuals and a host of other possibilities since AI is associated with vast fields of application.
There are various types of artificial intelligence such as reactive machines where their purpose is aiding a human interact with complex data, or assaults on the AI machine’s algorithms. However, all robots make mistakes for one reason or another and even though their degree of autonomy varies based on the functions they’re supposed to automise, it is essential to integrate them as one to avoid the chaos caused.
Learning Agents
Some authors examine learning agents as reflex agents that act without process which entirely depends on current perceptions and action of the moment. This agent can embed supervised, unsupervised or reinforcement learning depending on the task – making them ideal for areas where personalization is needed, for instance, advertising or virtual receptionist functions at e-commerce websites.
Trained AI systems exhibit a brain-like decision-making mechanism that processes all sensor inputs and determines the next course of action. A decision is made with regard to the factors influenced by the present environment, the rules determined in advance, or the experience acquired in the past. Because of this capability, learning AI is different from simple reflex agents, but quite suitable for more sophisticated, well-structured environments.
On the other hand, the increased complexity and resource requirements of holding an internal model can be a lot for developers to deal with. Additionally, an internal model that is held on to might be out of date or too specialized to deal with new situations that come up unexpectedly.
AI agents that learn and improve have an enhanced decision making mechanism that is similar to the one found in our brains. This enables the agents to consider all sensor information before determining the next possible action. The decision making considers the particular features of the surrounding environment, as well as certain rules or even memories based on previous actions when deploying the decision – it is this feature that makes learning AI quite different from simple reflex agents while also making them suitable for managing more complex well structured environments.
How to resolve failed to pull Helm chart
On the other hand, the additional management complexity and resources linked to the presence of an internal model may be overwhelming for the developers to bear. In addition, either an overly specific internal model or one that is out-of-date may find it difficult to generalize to new situations which are introduced haphazardly.
Learning agents can also be used to enhance operational efficiencies by identifying inefficiencies or areas that require enhancements making it a very appropriate resource for any e-commerce business aiming at providing tailored recommendations while reducing IT infrastructural costs by making efficient use of server resources.
AI agents have proved to be a revolutionary tool in automating the business processes and increasing productivity levels while at the same time bringing forth new levels of automation across all sectors. However, the effectiveness of this innovative tool depends on its algorithms designed to be complex and strong; most importantly, when applying this AI tool, it is important to consider such ethical aspects as data protection, bias prevention, transparency and compliance with the legal framework.